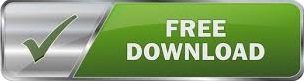

Multiscale distance matrix for fast plant leaf recognition. Hu, R., Jia, W., Ling, H., & Huang, D.Improving neural networks by preventing co-adaptation of feature detectors. E., Srivastava, N., Krizhevsky, A., Sutskever, I., & Salakhutdinov, R. A fast learning algorithm for deep belief nets. Early visual concept learning with unsupervised deep learning.Higgins, I., Matthey, L., Glorot, X., Pal, A., Uria, B., Blundell, C. The organization of behavior: A neuropsychological theory. In Proceedings of the IEEE conference on computer vision and pattern recognition (pp. Deep residual learning for image recognition. In Proceedings of the IEEE international conference on computer vision (pp. Delving deep into rectifiers: Surpassing human-level performance on imagenet classification. Deep learning for visual understanding: A review. Guo, Y., Liu, Y., Oerlemans, A., Lao, S., Wu, S., & Lew, M.In International Conference on Intelligent Computing (pp. Leaf recognition based on the combination of wavelet transform and gaussian interpolation. Goodfellow, I., Bengio, Y., Courville, A., & Bengio, Y. Foundations and Trends® in Signal Processing, 7(3–4), 197-387. Deeplab: Semantic image segmentation with deep convolutional nets, atrous convolution, and fully connected crfs. C., Papandreou, G., Kokkinos, I., Murphy, K., & Yuille, A. Plant leaf recognition using texture and shape features with neural classifiers. Chaki, J., Parekh, R., & Bhattacharya, S.Land use classification in remote sensing images by convolutional neural networks. Castelluccio, M., Poggi, G., Sansone, C., & Verdoliva, L.In European Conference on Computer Vision (pp. Searching the world’s herbaria: A system for visual identification of plant species. Indian Journal of Computer Science and Engineering, 2(2), 202-211. Content based leaf image retrieval (CBLIR) using shape, color and texture features. Computers and Electronics in Agriculture, 78(1), 9-18. Leaf classification in sunflower crops by computer vision and neural networks. Compressed Learning: A Deep Neural Network Approach. Automatic classification of plants based on their leaves. Burada yer alan görüntüler yansıma ve tersleme işlemleriyle 4 katına çıkarılmış yaklaşık olarak 7600 görüntü ile işlemler yapılmıştır.ĭerin öğrenme yöntemlerinden ise AlexNet, Vgg16, Vgg19, ResNet50, GoogleNet gibi derin öğrenme algoritmaları kullanılmış her bir algoritma için yaprak sınıflandırma uygulaması yapılarak, başarımları değerlendirilmiştir. Her bir görüntü sınıfı için ortalama 60 adet görüntü yer almaktadır.

Derin öğrenme yöntemlerinde, ön işlem ve özellik çıkarım gibi aşamalar Konvolüsyonel Sinir Ağları aracılığı ile gerçekleştirilmektedir.īu çalışmada, yaprak örüntüsü olarak kullanılan veri tabanında, görüntü örnekleri 32 sınıftan oluşan yaklaşık 1900 görüntü vardır. Derin öğrenme yöntemlerinde yapılan işlemlerde bu gibi işlemlere ihtiyaç duyulmamaktadır. Görüntü işleme yöntemleri ile yapılan sınıflandırma işlemlerinde ön işlem, özellik çıkarımı ve sınıflandırma yöntemi aracılığı ile sonuç alınmaktadır. In the context of the COVID-19 pandemic, we also seek articles discussing post-infectious degeneration in older adults.Bu makalede, bitki yapraklarının sınıflandırılması için kullanılan pek çok yönteme karşı derin öğrenme yoluyla sınıflandırılması yapılarak derin öğrenme algoritmalarının başarımları ortaya konulmuştur. In addition, we seek articles exploring the role of immunosenescence in age-related pathologies of the nervous system, including neurodegenerative and autoimmune disorders (e.g., AD, PD and MS), as well as the role of vascular disease in neurodegeneration. Therefore, we also welcome articles discussing the role of immune cells and cytokines for the ageing brain, as well as the role of inflammation in neuromuscular disorders associated with senescence. Over the last few decades, increasing evidence is implicating a role for the immune system in brain physiology and behaviour. We seek reviews, viewpoints, commentaries, or original research articles exploring the role of stress, mood disorders, and sensory systems during normal and premature immunosenescence. This topical collection will explore how complex neuroimmune interactions promote healthy and pathological ageing. Thematic Series: Neuroimmunology and Ageing
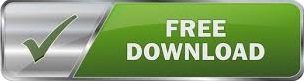